- #1
AcademicOverAnalysis
- 1
- 1
- TL;DR Summary
- The use of these theories in the data context is very limiting. They require continuous dynamics to be forward invariant, and the ultimate results are heuristic due to only supporting SOT convergence.
Hello everyone! This is my first post here. I am trying out an argument that I've been sculpting, and I thought this might be a good community where I can get some good feedback.
My work is in data driven methods for dynamical systems, and in particular, I am an operator theorist. I have been teaching a class on these methods at my university as a special topics course for graduate students, and I have posted all of my lectures up on YouTube at http://www.thatmaththing.com/. This include a lot of my original research, which has been aimed at improving Dynamic Mode Decomposition theory by removing a lot of constraints on dynamics that can be studied, as well as improving the convergence theory for DMD as well. You can find a playlist for the DMD lectures here.
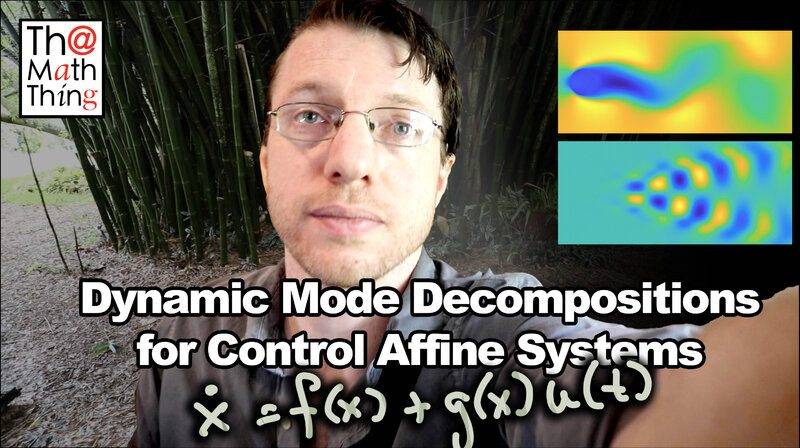
Most of these results have been published or are at least under review at conferences and journals. The image above links to a video related to a publication that will appear in the MTNS proceedings this fall. These give a completely different perspective on DMD than you'll find elsewhere, where we endeavor to clean up a lot of misconceptions in the field and remove a lot of unnecessary requirements on the dynamics.
In particular, if you look at the requirements on the dynamics from DMD, a data driven method, you will see that we immediately restrict ourselves to forward invariant systems. There are a lot of reasons for this. From the Koopman operator perspective, the discretization of a dynamical system yields holes in the discretization if the dynamics aren't forward invariant (usually verified by global Lipschitz conditions, but can also be shown with Lyapunov functions for certain systems). This means that we can't study simple nonlinear systems, such as \dot x = 1 + x^2, which gives tangent functions that have finite escape times.
Another source of the forward invariance comes from the desire to use Birkhoff and Von Neumann's Ergodic theorems. These require that we advance the trajectories to infinity and compute a limit to gain a fixed point of the Koopman operator. The use of these theorems come with their own problems, where the Von Neumann Ergodic Theorem works only on L^p spaces (p > 1) and only gives convergence with respect to equivalence classes of functions that agree almost everywhere. This is problematic, since members of L^p do not abide samples, which are sets of measure zero.
Our perspective is that DMD is best done over Reproducing Kernel Hilbert Spaces, where samples have well defined meaning for functions in that space, and there are available convergence theorems that demonstrate exactly how well a collection of samples describe a function (or observable). Moreover, we have removed the consideration of Koopman operators in light of Liouville operators, which are Koopman generators when the dynamics are forward invariant.
We have demonstrated that we can remove the forward invariance requirement when working with observed data. There is no reason that if we have a finite length trajectory that has been observed that I can't be used to sample the operator. We embed these trajectories in something we call occupation kernels, which are generalizations of occupation measures and are functions inside of a RKHS. This allows us to embed an entire trajectory within the Hilbert space, and we leverage the interactions between that function in the Hilbert space with Liouville operators to get approximations of the operator, and to develop a DMD routine for estimating the system state.
Moreover, by taking this perspective, removing Koopman operators and Ergodic theory, we can define these operators with differing domain and ranges, which will result in compact operators for the right choices of domains and ranges. This allows us to get norm convergence of our finite rank representations, which gives convergence DMD routines. This is a big advantage, where Koopman and Ergodic Theoretic methods can only achieve SOT convergence, which does not give guarantees for convergence of the spectra, and as DMD is a spectral method, this is a big problem.
This last statement is related to a manuscript we posted to arXiv recently, though the compactness results follow directly from some classical results in operator theory over function spaces. https://arxiv.org/abs/2106.02639
So long story short, we have been able to remove Koopman operators from the analysis, and we lean on sampling theory to make convergent DMD algorithms. I know that there is a long history of intertwining Koopman operators and Ergodic Theory with DMD analysis, so I am looking for input from the community here :)
My work is in data driven methods for dynamical systems, and in particular, I am an operator theorist. I have been teaching a class on these methods at my university as a special topics course for graduate students, and I have posted all of my lectures up on YouTube at http://www.thatmaththing.com/. This include a lot of my original research, which has been aimed at improving Dynamic Mode Decomposition theory by removing a lot of constraints on dynamics that can be studied, as well as improving the convergence theory for DMD as well. You can find a playlist for the DMD lectures here.
Most of these results have been published or are at least under review at conferences and journals. The image above links to a video related to a publication that will appear in the MTNS proceedings this fall. These give a completely different perspective on DMD than you'll find elsewhere, where we endeavor to clean up a lot of misconceptions in the field and remove a lot of unnecessary requirements on the dynamics.
In particular, if you look at the requirements on the dynamics from DMD, a data driven method, you will see that we immediately restrict ourselves to forward invariant systems. There are a lot of reasons for this. From the Koopman operator perspective, the discretization of a dynamical system yields holes in the discretization if the dynamics aren't forward invariant (usually verified by global Lipschitz conditions, but can also be shown with Lyapunov functions for certain systems). This means that we can't study simple nonlinear systems, such as \dot x = 1 + x^2, which gives tangent functions that have finite escape times.
Another source of the forward invariance comes from the desire to use Birkhoff and Von Neumann's Ergodic theorems. These require that we advance the trajectories to infinity and compute a limit to gain a fixed point of the Koopman operator. The use of these theorems come with their own problems, where the Von Neumann Ergodic Theorem works only on L^p spaces (p > 1) and only gives convergence with respect to equivalence classes of functions that agree almost everywhere. This is problematic, since members of L^p do not abide samples, which are sets of measure zero.
Our perspective is that DMD is best done over Reproducing Kernel Hilbert Spaces, where samples have well defined meaning for functions in that space, and there are available convergence theorems that demonstrate exactly how well a collection of samples describe a function (or observable). Moreover, we have removed the consideration of Koopman operators in light of Liouville operators, which are Koopman generators when the dynamics are forward invariant.
Moreover, by taking this perspective, removing Koopman operators and Ergodic theory, we can define these operators with differing domain and ranges, which will result in compact operators for the right choices of domains and ranges. This allows us to get norm convergence of our finite rank representations, which gives convergence DMD routines. This is a big advantage, where Koopman and Ergodic Theoretic methods can only achieve SOT convergence, which does not give guarantees for convergence of the spectra, and as DMD is a spectral method, this is a big problem.
This last statement is related to a manuscript we posted to arXiv recently, though the compactness results follow directly from some classical results in operator theory over function spaces. https://arxiv.org/abs/2106.02639
So long story short, we have been able to remove Koopman operators from the analysis, and we lean on sampling theory to make convergent DMD algorithms. I know that there is a long history of intertwining Koopman operators and Ergodic Theory with DMD analysis, so I am looking for input from the community here :)